Friday, November 22, 2024
Driving Innovation in Drug Discovery: The Role of ML Competitions

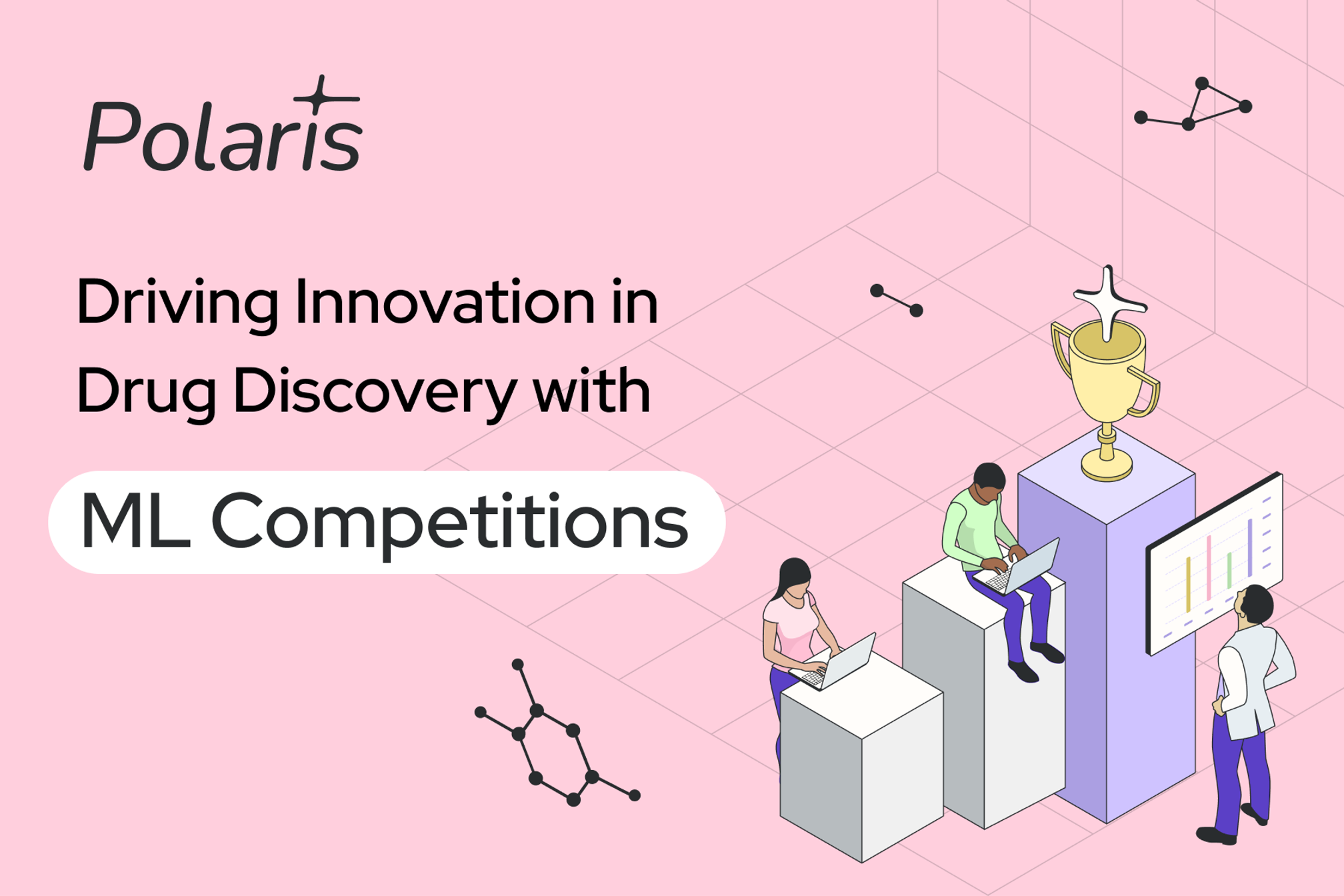
In machine learning, progress relies heavily on the ability to measure performance and benchmarks are the foundation of this measurement. They help us quantify improvements, compare models, and ultimately drive innovation. Competitions are a slightly different spin on benchmarks. These are prospective benchmarks that often involve the release of new data around well-defined tasks aligned with real-world applications.
While fields like natural language processing (NLP) and computer vision (CV) have long benefitted from standardized benchmarks and well-organized competitions, ML for drug discovery (MLDD) faces unique challenges. What can we learn from other fields and what does the current landscape of competitions look like in MLDD?
Retrospective vs. prospective benchmarks
Before we get into competitions, let’s start by defining the two different types of benchmarks. They can be broadly organized into two categories: retrospective or prospective (competitions).
Retrospective Benchmarks
Retrospective benchmarks have a fixed dataset and evaluation criteria that are easily accessible and provide a replicable environment for testing models (in an ideal world, but this is not often the case 😅).
While there’s value in retrospective benchmarks, they come with inherent limitations. Most researchers have good intentions. They use the test set as little as possible and try to minimize leakage. Over time, these benchmarks are studied extensively. Experiments using the same test set are run again and again, slowly biasing the model and results. Now, models that seemingly rank 1st on a given leaderboard struggle in real-world situations because they’re biased to the training set and cannot generalize well across new data.
Read the latest pre-print from the Small Molecule Steering Committee if you’re interested in learning more about the replicability crisis in ML-based science.
Competitions
In an ideal world, you use a test set once to avoid biasing the results. But this is very unrealistic for retrospective benchmarks, and is where competitions come in. Typically, with competitions, there’s newly generated data specifically for well-defined tasks that are aligned with real-world applications. You’ll only see the final results for just one of your submissions and the test set is only used once. This provides researchers with the unique opportunity to get an unbiased estimate of prospective performance.Over the years, there have been countless examples demonstrating how competitions are instrumental in driving progress across various fields. A classic example is the ImageNet Large Scale Visual Recognition Challenge (ILSVRC) for computer vision. ImageNet not only set a high bar for model performance but also drove the development of groundbreaking architectures like AlexNet.
In drug discovery, most people are familiar with the Critical Assessment of Structure Prediction (CASP). With regular competitions and rigorously evaluated results, it became a driving force behind the advancements in computational biology that the field is benefiting from today. CASP is where AlphaFold first entered the scene. Eventually, we saw AlphaFold reach AlexNet-like performance gains in protein structure prediction in 2018. Since then, the field has advanced rapidly, culminating in the 2024 Nobel Prize in Chemistry being awarded to the creators of AlphaFold. This has been an exciting turning point in the community, marking the beginning of a new era in TechBio.
Other competitions covering different ML applications in drug discovery include:
- Critical Assessment of Computational Hit-finding Experiments (CACHE) challenges, aiming to improve hit identification methods, and;
- D3R Grand Challenge for protein-ligand docking and binding affinity prediction.
Competitions in 2024 and beyond
It feels like 2024 has been a banner year for competitions, with several organizations contributing new datasets and challenges:
- Leash Bio hosted a competition around their Big Encoded Library for Chemical Assessment Dataset (BELKA) dataset. Their team tested some 133M small molecules for their ability to interact with 1 of 3 protein targets via DNA-encoded library technology. The dataset contains ~4.25 billion physical measurements (that’s bigger than PubChem bioactivities, DrugBank, ChEMBL, and 1000x bigger than BindingDB).
- Adaptv Bio hosted 2 rounds of their protein design competition where researchers can submit designs for a binding protein to EGFR. Round 1 saw over 700 submissions, with 200 being synthesized in the lab. In Round 2, the newly generated data was released to the community (along with a benchmark) and designers were given another chance to submit their designs. With over 1,000 submissions, 400 proteins are currently being synthesized and tested experimentally. The results and new data will be released on December 4th!
- On the topic of protein design competitions, there was also the BioML challenge organized by the University of Texas and the Protein Engineering Tournament from the team at Align to Innovate, which recently received $2M in funding to scale this effort in 2025.
While competitions in drug discovery have historically been constrained by the high costs and time required to generate data, it's encouraging to see a growing number of organizations stepping up to provide valuable datasets for the community.
- The ASAP Discovery Consortium has released large quantities of molecular and target structure data (with more to come, stay tuned 👀).
- The OpenADMET consortium, with OMSF, John Chodera, UCSF, and others, was awarded a $30M+ grant to develop a comprehensive open library of experimental and structural datasets for therapeutic development.
- Companies like Recursion, which previously released rxrx.ai, a collection of phenomics datasets, recently released RxRx3-core, a challenge dataset that includes labelled images of 735 genetic knockouts and 1,674 small-molecule perturbations. This was released along with OpenPhenom-S/16, the first of potentially more open-source foundation models for microscopy data.
What does this mean for Polaris?
At Polaris, our mission is to bring innovators and practitioners closer together to develop methods that matter. One of the ways in which we’re doing so is by forming industry steering committees around specific modalities. Starting with a focus on small molecule predictive modeling tasks, we’ve already released guidelines on method comparison, with more resources to come on splitting methods and data curation.
We’ve also been heads down building the infrastructure for the Polaris Hub. We aim to support any drug discovery-related dataset or benchmark, no matter the size or modality you’re working with. Competitions are a key part of this vision and we aim to make it easier for researchers to host challenges and share new data with the community.If you’re looking to release new data or benchmarks to the community and are interested in doing so through some sort of competition or blind challenge, reach out! We’d love to collaborate to bring more resources to the community.