Small Molecule Guidelines
Crafted by an industry steering committee. Read the guidelines on
method evaluation, method comparison, and dataset curation.
The Nuances of Benchmarking
Recognizing the unique challenges of applying ML to small-molecule, predictive modeling tasks in drug discovery—such as complex, limited datasets and the need for interdisciplinary expertise—we formed an industry steering committee to develop comprehensive guidelines and resources for the community.
By pooling insights from decades of experience, our steering committee aims to set new standards for method evaluation (e.g., dataset splitting, evaluation metrics), method comparison (e.g., statistical tests), and data curation.
We started with a call to action. In our letter, we outline common pitfalls and challenges in benchmarking that contribute to a growing gap between ML innovations and their practical impact on drug discovery programs. We believe that an open-science, cross-industry, and interdisciplinary effort is a crucial first step toward addressing these challenges, and we invite other experts to join us.
Read The LetterMeet the Steering Committee
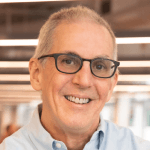
Pat Walters
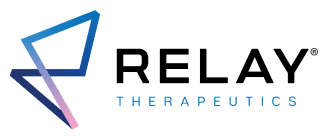
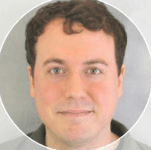
Jeremy Ash
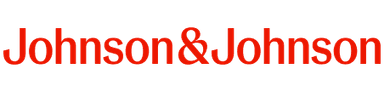
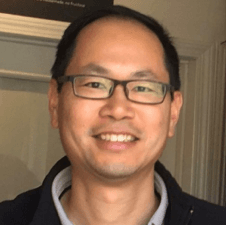
Alan Cheng
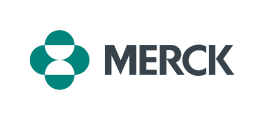
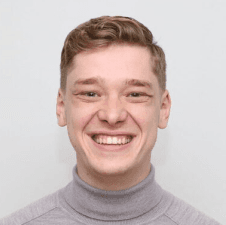
Cas Wognum
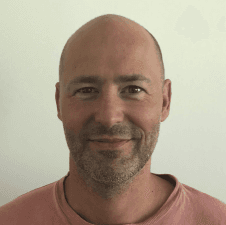
Djork-Arné Clevert
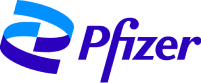
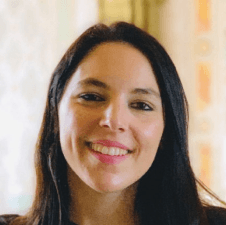
Raquel RodrÃguez-Pérez
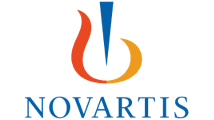
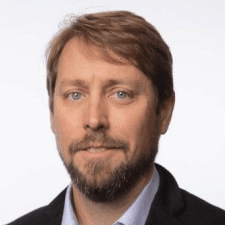
Daniel Price
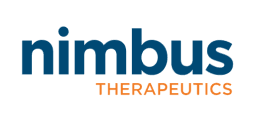
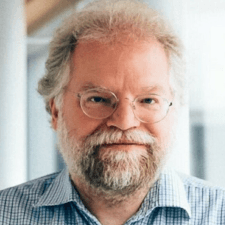
Ola Engkvist
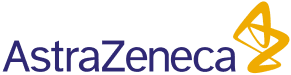
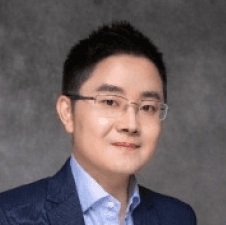
Cheng Fang
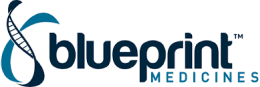
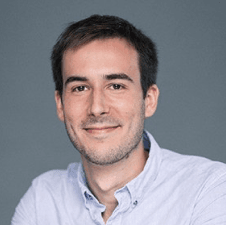
Matteo Aldeghi
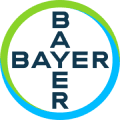
Publication Timeline
This is what we’re starting with. Have some ideas? Let us know!
Method Comparison
To contextualize the results of a new ML method, its performance is typically compared to the state of the art and baselines. This paper proposes guidelines for small-molecule, predictive modeling on how to do this comparison in a robust way such that you can expect your conclusions to generalize to similar datasets and real-world use cases.
Read the PaperSplitting Methods
To prevent models from merely memorizing training data—a problem known as overfitting — it's crucial to ensure that the similarity between training and test sets reflects real-world applications. This paper provides guidelines for small-molecule predictive modeling on how to measure generalization in a way that aligns with practical use cases.
Data Curation
Large industrial datasets are rarely published due to competitive and intellectual property concerns. Therefore, drug discovery benchmarks often rely on public databases like ChEMBL. Curating datasets from these sources requires deep expertise in data generation processes and data modalities. To address this challenge, we propose guidelines for curating small-molecule datasets.
We Want Your Feedback
Want updates on the guidelines?
Sign up to get notified.
We care about your data. Read our privacy policy.